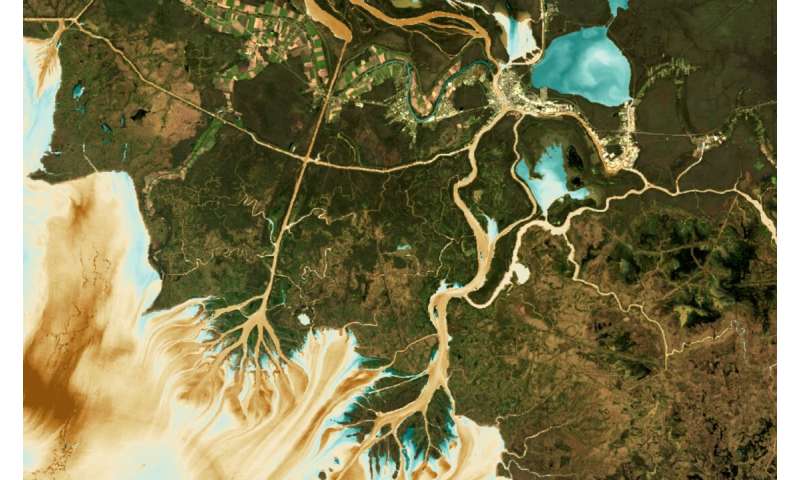
This visual shows how AquaSat can be used to predict Total Suspended Sediment in the Wax Lake and Atchafalaya Deltas, where brown colors are high sediment concentrations and blue values are low. Credit: Matthew Ross/Colorado State University
Access to abundant, clean, water for drinking, recreation and the environment is one of the 21st century's most pressing issues. Directly monitoring threats to the quality of fresh water is critically important, but because current methods are costly and not standardized, comprehensive water quality datasets are rare. In the United States, one of the most data-rich countries in the world, fewer than 1% of all bodies of fresh water have ever been sampled for quality.
In a new paper, AquaSat: a dataset to enable remote sensing of water quality for inland waters, a team led by Colorado State University Assistant Professor Matt Ross matched large public datasets of water quality observations with satellite imagery to address the challenges of measuring water quality efficiently and cost-effectively.
Threats we can't fully understand—yet
According to Ross, a watershed scientist in the Department of Ecosystem Science and Sustainability, there are many threats to water quality, including nutrients from agricultural runoff that support algae blooms; sedimentation in reservoirs that cause distribution challenges; and dissolved carbon from decaying leaves that interrupts chemical reactions that keep water clean and safe for drinking.
For the most part, government entities monitor water quality in the U.S. by sending scientists into the field to measure variables like the amount of chlorophyll (from algae), concentrations of suspended sediment, dissolved organic carbon, and water clarity in person.
But, as Ross and his team explain, to fully understand and inventory changes in water quality, a far larger dataset is required; that in turn requires more and more people to do field sampling, which is very expensive and unlikely to completely address the problem.
Instead, the team suggests using remote sensing from satellite imagery could be a way to vastly expand our understanding of variation in water quality at continental scales, with little extra cost for sampling.

"Satellites have fundamentally changed how we understand long-term changes in agriculture, forests, fires and other land cover changes," explained CSU's Matt Ross. "However, there has been less use of the Landsat archive for understanding inland water quality changes." Credit: Matthew Ross/Colorado State University
Merging satellite imagery with field measurements
For many decades, scientists have known that water's color tells us something about what is in it. Bright tan water likely indicates a river full of sediment. Green swirls over Lake Erie show algae growing and producing chlorophyll. Dark brown waters draining tannin-rich forests and swamps turn blue waters into a tea-colored brown because of how light interacts with certain dissolved organic carbon compounds.
Imaging satellites orbiting the earth, including Landsat, detect these color variations as they take images of the Earth every 16 days.
"These satellites have fundamentally changed how we understand long-term changes in agriculture, forests, fires, and other land cover changes," explained Ross. "However, there has been less use of the Landsat archive for understanding inland water quality changes."
One challenge of using Landsat images to evaluate water quality is the lack of a centralized dataset that pairs the satellite imagery with on-the-ground observations. These matchups—for example, when satellites snap a picture on the same day someone takes an algae sample—can be used to build algorithms that use imagery alone to predict water quality from space.
Fewer than 1,000 such matchups, mostly built for individual studies, currently exist, slowing researchers' ability to build, test, and apply large-scale models to predict water quality for every cloud-free image in the Landsat archive.
A 'symphony of data'
The CSU researchers built a novel dataset of more than 600,000 matchups between water quality field measurements and Landsat imagery, creating what Ross calls a "symphony of data."
The water quality data came from two public sources: the Water Quality Portal, a federal data clearinghouse from more than 400 different state, local, and federal agencies; and LAGOS-NE, an open-science dataset of lake water quality measurements for the Northeastern United States. Combined, these datasets provide more than 6 million water quality observations.
Using open-source software and Google Earth Engine, the authors merged the water quality data with the Landsat archive from 1984-2019. Both the raw datasets and the merged matchup dataset, which they call AquaSat, are now available along with the underlying code so future users can update, change, and improve it.
The authors expect that this dataset will unlock powerful new applications in remote sensing of water quality.
"We're hoping these tools will help build national-scale water quality estimates for large rivers and lakes," said Ross. "These data would dramatically improve our understanding of water quality change at the macro-scale and allow the remote sensing community to compare methods and collectively improve our approach."
In the future, Ross's team expects to go beyond the U.S. to employ these same methods to improve water quality monitoring in other places with little or no field observations.
More information:
Matthew R. V. Ross et al, AquaSat: A Data Set to Enable Remote Sensing of Water Quality for Inland Waters, Water Resources Research (2019). DOI: 10.1029/2019WR024883
Citation:
Studying water quality with satellites and public data (2019, December 4)
retrieved 4 December 2019
from https://phys.org/news/2019-12-quality-satellites.html
This document is subject to copyright. Apart from any fair dealing for the purpose of private study or research, no
part may be reproduced without the written permission. The content is provided for information purposes only.
#Environment | https://sciencespies.com/environment/studying-water-quality-with-satellites-and-public-data/
No comments:
Post a Comment